Annual Showcase 2025
Empowering Machine Learning Collaboration Through Remote Sharing Platforms
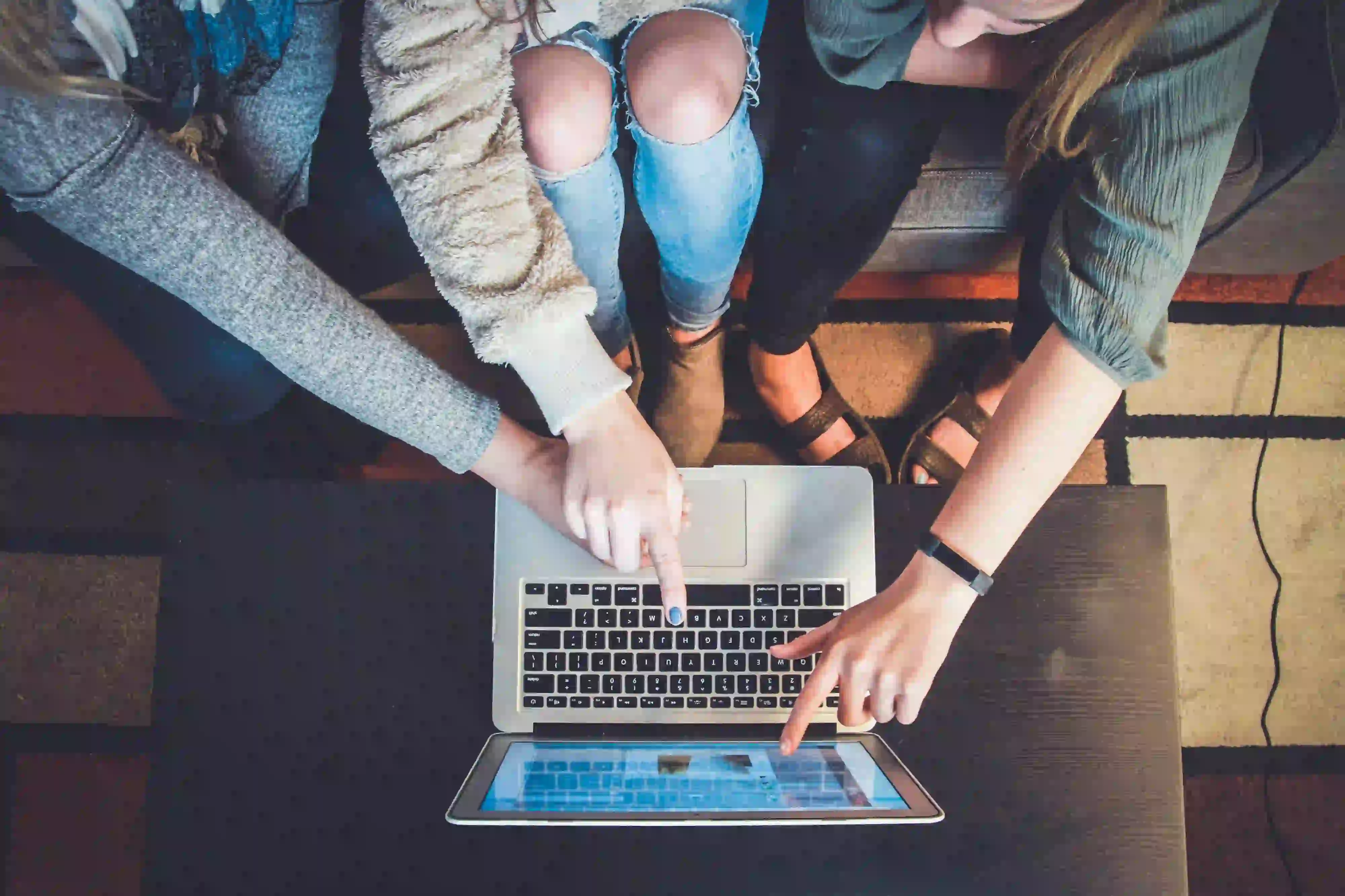
The Machine Learning module in BUPT Beijing, Principles of Machine Learning in Hainan and London has provided an exciting and collaborative experience for students across three locations: BUPT Beijing, BUPT Hainan, and QMUL London. This unique initiative not only challenged students to apply fundamental machine learning principles but also introduced an innovative co-created dataset approach, emphasizing the power of data sharing and interdisciplinary collaboration through an advanced **remote sharing platform.
A Shared Learning Experience
For this project, students from BUPT Beijing, BUPT Hainan, and QMUL London worked on the same mini-project while following a unified scoring system. The biggest highlight of this activity was the emphasis on collaborative dataset creation, an approach that enabled students to actively contribute to a publicly available research dataset, fostering a sense of ownership and real-world impact. With around 900 students participating, this initiative has demonstrated the scalability and effectiveness of remote collaboration in machine learning education.
The MLEnd Deception Dataset: A Collaborative Effort
As part of the project, students contributed to the development of the MLEnd Deception Dataset, a large dataset containing over 3,000 data entries of truthful and deceptive stories recorded in both English and students' native languages. Each participant recorded six storytelling audio files:
- Three truthful stories (two in English, one in their native language)
- Three deceptive stories (two in English, one in their native language)
To ensure ethical data handling, students were provided with consent forms before contributing their recordings. This allowed them to make informed decisions regarding the use of their data. All audio recordings were anonymized and will be made publicly accessible to facilitate further research in machine learning and linguistic analysis.
Working with Voice Data: From Problem to Solution
Once the dataset was created, students explored various aspects of voice data analysis and machine learning techniques to identify patterns in deceptive versus truthful storytelling. This stage of the project required students to define a research problem based on the dataset and apply machine learning models to address it. Some key focus areas included:
- Feature extraction from audio recordings
- Classification techniques for deception detection
- Sentiment and emotion analysis in storytelling
A Great Collaboration Across Universities and Locations
This mini-project was a significant collaborative effort involving two universities across three locations—BUPT Beijing, BUPT Hainan, and QMUL London. It showcased the effectiveness of the remote sharing platform, proving that students from different locations could work together seamlessly, contributing to a large-scale project despite geographical distances. This initiative highlights the potential of international collaboration in advancing machine learning research and education.
Interdisciplinary Collaboration with Linguists
An exciting addition to this year’s project was the involvement of linguists, who provided valuable insights into speech patterns, emotional cues, and linguistic differences between truthful and deceptive narratives. This interdisciplinary approach enhanced the learning experience, allowing students to integrate both technical (machine learning) and human-centric (linguistic) perspectives in their research.
Introducing the Machine Learning Competition
To further challenge students and encourage deeper engagement, we initiated a machine learning competition across all participating locations. This competition encouraged students to explore more complex research problems, push the boundaries of their understanding, and develop innovative solutions beyond the mini-project. By participating, students were motivated to apply their skills in a competitive yet collaborative environment, fostering creativity and problem-solving abilities.
Engaged and Collaborative Learning
Throughout the project, students demonstrated exceptional engagement and teamwork. They actively shared knowledge, helped each other troubleshoot issues, and collaborated on ideas, making this initiative a true reflection of collective learning. The co-creation of the dataset not only enhanced their understanding of machine learning but also provided a meaningful contribution to the research community.
Looking Ahead
The Machine Learning module across BUPT Beijing, Hainan, and London has once again showcased the value of collaborative learning and research-driven education. Looking forward, we aim to expand this initiative by including more modules and projects to further empower the remote sharing platform, making it an even more powerful tool for international collaboration in education and research.
A huge thank you to all students, faculty members, and linguists who contributed to this initiative! A special thanks to Nikesh and Jesus for their great support and contribution to making this project a success. Stay tuned for future research collaborations and projects in the field of machine learning.
For more information on our programs and research activities, visit our official website: [https://www.qmul.ac.uk/eecs/china/jsic/](https://www.qmul.ac.uk/eecs/china/jsic/).