Dr Nicolás Hernández
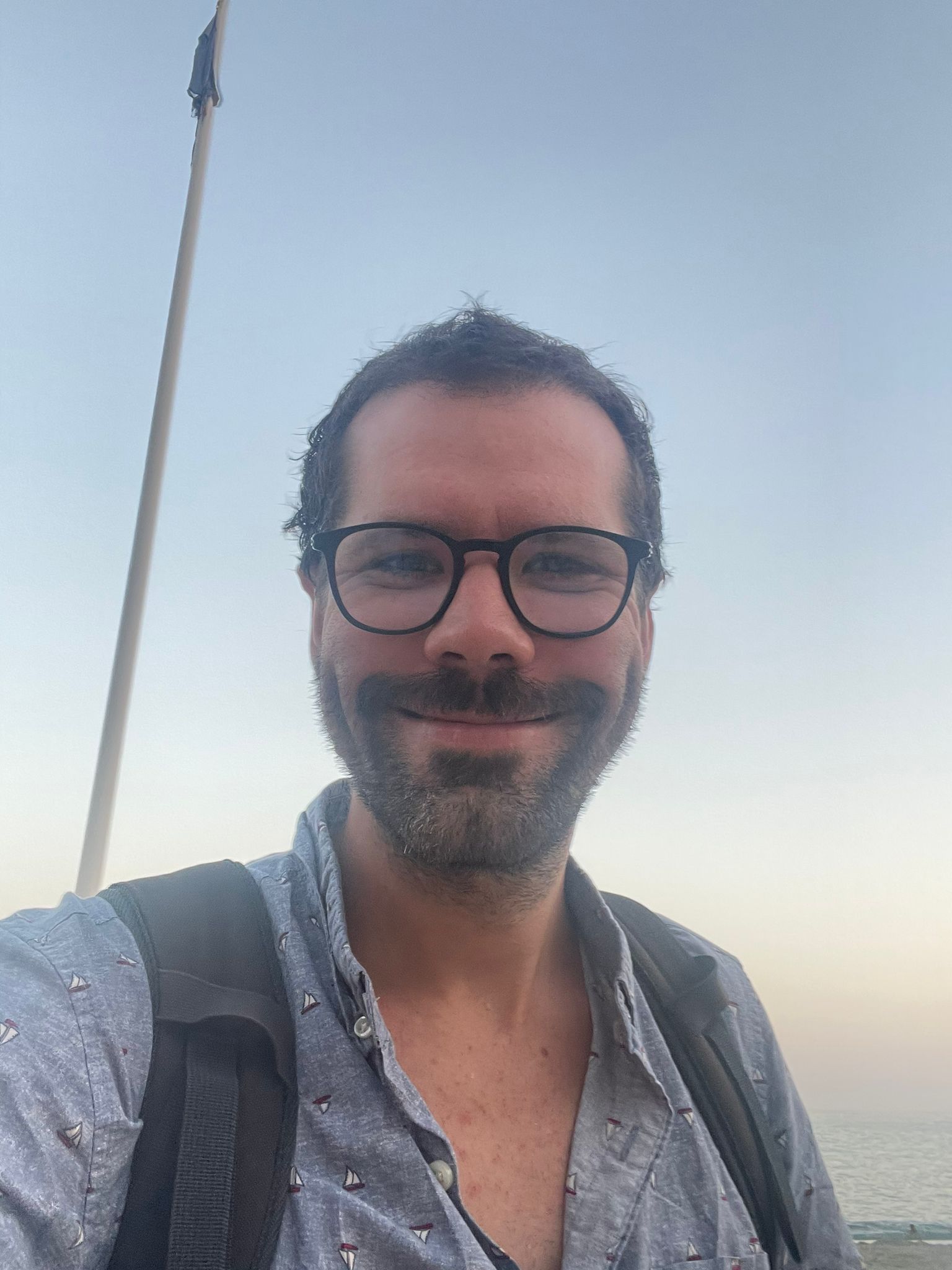
Lecturer in Statistics
Email: n.hernandez@qmul.ac.ukRoom Number: Mathematical Sciences Building, Room: MB-125Website: https://nicolashernandezb.github.io/Office Hours: School Social Hub, MB-B11, Thursdays 11:30 to 12:30.
Profile
Dr. Hernández is a Lecturer in Statistics within the Data Science, Statistics and Probability Centre at the School of Mathematical Sciences. He joined QMUL after spending 2 years as a Senior Research Fellow within the Institute of Mathematics and Statistical Science at the Department of Statistical Science, UCL. Previously he was appointed as a PDRA at the MRC Biostatistics Unit of the University of Cambridge. Before that he completed his PhD studied about ‘‘Statistical learning methods for functional data with applications to prediction, classification and outlier detection’’ at the Department of Statistics of Universidad Carlos III de Madrid.
His main research is oriented to develop statistical and machine learning methods to tackle inferential problems in high-dimensional and functional data over different fields such as: energy, economics, the environment, demography, business, finance, health and genetics. He has mainly focused on predictive confidence bands for functional time series; domain selection and classification in the Functional Data context; and outlier detection for stochastic processes using Information Theory tools.
Teaching
Module Leader
Biostatistics and Medical Statistics at MSc in Applied Statistics and Data Science.
This module will start by providing an overview of the field and its contemporaneous challenges with particular emphasis on ethical considerations and data confidentiality related to biomedical research.
Students will also be provided with a review of the basic probabilistic and statistical techniques such as the basic probability distributions and hypothesis testing.
The rest of the module will combine coverage of the following:
1. Statistical notions
Including analysis of categorical data (chi-square, logistic regression) and continuous data (t-test, ANOVA)
2. Applications
Clinical trials, survival analysis, causal inference, meta-analysis and systematic reviews.
IT Labs Instructor
Time Series Analysis for Business at MSc in Business Analytics
This module will present methods for time series analysis.These will allow the student to understand better how to use and extract information from historical business data series. In particular, the student will learn how to extract the pivotal concepts of time series data, including the trend and cyclic components of a data series, calculate the autocorrelation, learn about autoregressive and moving average models, and cointegration. The module will develop the notions around realistic business examples and an implementation of the methods will be provided using the statistic software R.